目录
- 前言
- 一、快速开始
- 1-1、介绍
- 1-2、安装
- 1-3、开发你自己的Agent
- 二、Qwen-Agent的使用和开发过程
- 2-1、Agent
- 2-1-1、Agent使用
- 2-1-2、Agent开发
- 2-2、Tool
- 2-2-1、工具使用
- 2-2-2、工具开发
- 2-3、LLM
- 2-3-1、LLM使用
- 2-3-2、LLM开发
- 三、基于Qwen-Agent的案例分析
- 3-1、
- 3-2、
- 总结
前言
Qwen-Agent是一个开发框架。开发者可基于本框架开发Agent应用,充分利用基于通义千问模型(Qwen)的指令遵循、工具使用、规划、记忆能力。本框架也提供了浏览器助手、代码解释器、自定义助手等示例应用。
一、快速开始
1-1、介绍
Qwen-Agent: 是一个开发框架。开发者可基于本框架开发Agent应用,充分利用基于通义千问模型(Qwen)的指令遵循、工具使用、规划、记忆能力。本项目也提供了浏览器助手、代码解释器、自定义助手等示例应用。
1-2、安装
1、使用pip安装:
pip install -U qwen-agent
2、从Github安装最新版本
git clone https://github.com/QwenLM/Qwen-Agent.git
cd Qwen-Agent
pip install -e ./
1-3、开发你自己的Agent
概述:下面的示例说明了创建一个能够读取PDF文件和利用工具的代理的过程,以及构建自定义工具,以下为详细介绍:
- 添加一个自定义工具:图片生成工具
- 使用到的LLM模型配置。
- 创建Agent,这里我们以“Assistant”代理为例,它能够使用工具和读取文件。
- 以聊天机器人的形式运行助理。
import pprint
import urllib.parse
import json5
from qwen_agent.agents import Assistant
from qwen_agent.tools.base import BaseTool, register_tool# Step 1 (Optional): Add a custom tool named `my_image_gen`.
@register_tool('my_image_gen')
class MyImageGen(BaseTool):# The `description` tells the agent the functionality of this tool.description = 'AI painting (image generation) service, input text description, and return the image URL drawn based on text information.'# The `parameters` tell the agent what input parameters the tool has.parameters = [{'name': 'prompt','type': 'string','description': 'Detailed description of the desired image content, in English','required': True}]def call(self, params: str, **kwargs) -> str:# `params` are the arguments generated by the LLM agent.prompt = json5.loads(params)['prompt']# 对提示词进行URL编码prompt = urllib.parse.quote(prompt)# return json5.dumps({'image_url': f'https://image.pollinations.ai/prompt/{prompt}'},ensure_ascii=False)# Step 2: Configure the LLM you are using.
# 这里是需要配置模型的地方。需要填写模型名字,以及model_server,即模型所在服务器名字,如果没有,也可以考虑使用api_key。
llm_cfg = {# Use the model service provided by DashScope:# model:模型名称# model_server:模型所在的服务器# api_key: 所使用到的api-key,可以显示的设置,也可以从环境变量中获取'model': 'qwen-max','model_server': 'dashscope',# 'api_key': 'YOUR_DASHSCOPE_API_KEY',# It will use the `DASHSCOPE_API_KEY' environment variable if 'api_key' is not set here.# Use a model service compatible with the OpenAI API, such as vLLM or Ollama:# 'model': 'Qwen1.5-7B-Chat',# 'model_server': 'http://localhost:8000/v1', # base_url, also known as api_base# 'api_key': 'EMPTY',# (Optional) LLM hyperparameters for generation:# 用于调整生成参数的可选配置'generate_cfg': {'top_p': 0.8}
}# Step 3: Create an agent. Here we use the `Assistant` agent as an example, which is capable of using tools and reading files.# agent的提示词指令
system_instruction = '''You are a helpful assistant.
After receiving the user's request, you should:
- first draw an image and obtain the image url,
- then run code `request.get(image_url)` to download the image,
- and finally select an image operation from the given document to process the image.
Please show the image using `plt.show()`.'''# 工具列表,指定Assistant可以访问的工具,一个是自定义的工具,一个是代码执行器
tools = ['my_image_gen', 'code_interpreter'] # `code_interpreter` is a built-in tool for executing code.
# 助理可以读取的文件路径
files = ['./examples/resource/doc.pdf'] # Give the bot a PDF file to read.# 初始化Assistant
bot = Assistant(llm=llm_cfg,system_message=system_instruction,function_list=tools,files=files)# Step 4: Run the agent as a chatbot.
messages = [] # This stores the chat history.
while True:# For example, enter the query "draw a dog and rotate it 90 degrees".query = input('user query: ')# Append the user query to the chat history.messages.append({'role': 'user', 'content': query})response = []for response in bot.run(messages=messages):# Streaming output.print('bot response:')pprint.pprint(response, indent=2)# Append the bot responses to the chat history.messages.extend(response)
-
首先输入任务目标:draw a dog and rotate it 90 degrees
-
绘制的狗子图片:
-
结果输出:
-
Agent处理后的狗子图片展示:
二、Qwen-Agent的使用和开发过程
2-1、Agent
2-1-1、Agent使用
概述: Qwen-Agent的Agent类集成了工具调用和LLM的接口,不同Agent类的工作流不同。
Qwen-Agent提供一个通用的Agent类,Assistant类,可以处理大多数任务,例如:
- 它支持角色扮演
- 它提供了自动规划和工具调用能力
- 它接受文档输入,并且可以使用集成的RAG策略来解析文档。
案例分析:
import os
from qwen_agent.agents import Assistant
llm_cfg = {'model': 'qwen-max'}
tools = ['image_gen', 'amap_weather'] # image_gen and code_interpreter is a built-in tool in Qwen-Agent
system = 'According to the user\'s request, you first draw a picture and then automatically run code to download the picture ' + \'and select an image operation from the given document to process the image'bot = Assistant(llm=llm_cfg,system_message=system,function_list=tools,files=[os.path.abspath('doc.pdf')])messages = [{'role': 'user', 'content': 'a cute cat'}]
for response in bot.run(messages=messages):print('bot response:', response)"""Running results:
[{'role': 'assistant', 'content': '', 'function_call': {'name': 'my_image_gen', 'arguments': '{"prompt": "a cute cat"}'}},{'role': 'function', 'content': '{"image_url": "https://image.pollinations.ai/prompt/a%20cute%20cat"}', 'name': 'my_image_gen'},{'role': 'assistant', 'content': "Here is the image of a cute cat based on your description:\n\n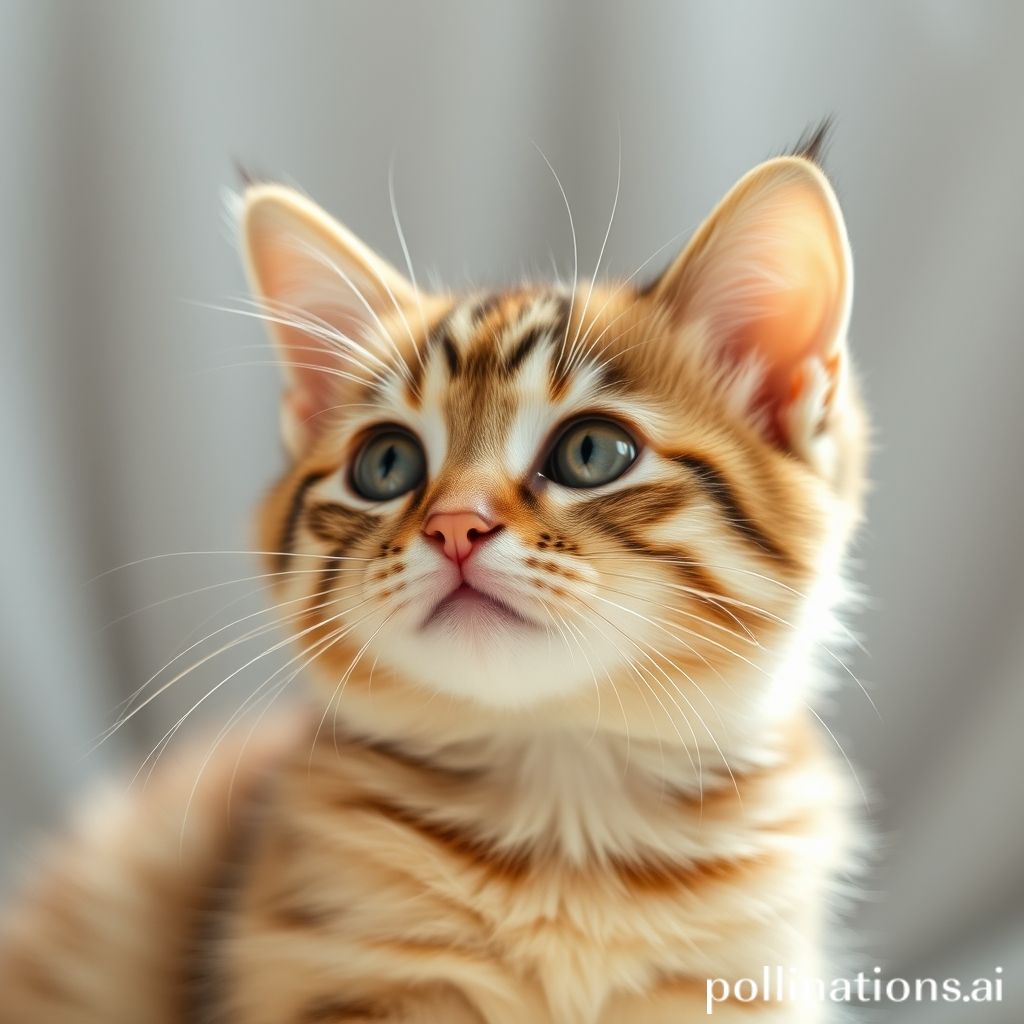\n\nNow, let's proceed with downloading this image and performing an operation such as flipping it."},{'role': 'assistant', 'content': '', 'function_call': {'name': 'code_interpreter', 'arguments': '```py\nfrom PIL import Image\nimport requests\n\n# Downloading the cute cat image\nurl = "https://image.pollinations.ai/prompt/a%20cute%20cat"\nresponse = requests.get(url)\nwith open("cute_cat.jpg", \'wb\') as file:\n file.write(response.content)\n\n# Flipping the image horizontally\ndef flip_image_horizontally(filename):\n img = Image.open(filename)\n flipped_img = img.transpose(Image.FLIP_LEFT_RIGHT)\n flipped_img.save("flipped_cute_cat.jpg")\n return flipped_img\n\n# Performing the horizontal flip\nflipped_cat = flip_image_horizontally("cute_cat.jpg")\n```'}},{'role': 'function', 'content': 'Finished execution.', 'name': 'code_interpreter'},{'role': 'assistant', 'content': 'The image of the cute cat has been downloaded and flipped horizontally. The flipped image has been saved as "flipped_cute_cat.jpg". Since we\'re in a text-based environment, I can\'t display the actual image here, but you can check it out at the location where the script was executed.'}
]
"""
此外我们还提供了一个通用的多代理类:GroupChat类。这个类管理一个agent列表并自动维护它们的语音顺序。这个类的特点包括:
- 在接收到外部输入后,它自动协调内置代理的说话顺序,并按顺序将它们的响应返回给用户;
- Human-in-the-loop:用户也被定义为Agent,群聊可以在必要时请求用户的反馈;
- 用户可以随时中断群聊。
2-1-2、Agent开发
Demo1: 自定义Agent,一个用于可视化故事讲解的代理,它结合了图像理解和文章撰写的能力,将多模型、多工具的能力集成到一个工作流中。嵌套使用多个Agent。Agent介绍如下:
- image_agent:使用Qwen-VL理解图片内容
- writing_agent:帮助撰写作文
工作流程以及代码实现:
- 用户输入:用户提供一组包含图像的消息
- 图像理解:image-agent 使用qwen-vl-max模型,对图像进行详细描述
- 撰写文章:根据图像描述,结合知识库撰写完整的叙事故事。
- 生成结果:最终响应将包括图像描述和叙事故事。
import copy
from typing import Dict, Iterator, List, Optional, Union# 代理类
from qwen_agent import Agent
# 助理类
from qwen_agent.agents import Assistant
# 基础聊天模型
from qwen_agent.llm import BaseChatModel
#
from qwen_agent.llm.schema import ContentItem, Message
from qwen_agent.tools import BaseTool# 继承自Agent基类
class VisualStorytelling(Agent):"""Customize an agent for writing story from pictures"""# function_list:可以使用的工具列表,默认为空。# llm:指定的语言模型,默认为空。def __init__(self,function_list: Optional[List[Union[str, Dict,BaseTool]]] = None,llm: Optional[Union[Dict, BaseChatModel]] = None):super().__init__(llm=llm)# Nest one vl assistant for image understanding# 初始化视觉理解Agentself.image_agent = Assistant(llm={'model': 'qwen-vl-max'})# Nest one assistant for article writing# 初始化写作Agent# 提供指定工具和文件,用于支持写作任务。self.writing_agent = Assistant(llm=self.llm,function_list=function_list,system_message='You are a student, first, you need to understand the content of the picture,' +'then you should refer to the knowledge base and write a narrative essay of 800 words based on the picture.',files=['https://www.jianshu.com/p/cdf82ff33ef8'])# 定义工作流程,用于处理一组输入消息,生成视觉故事。def _run(self,messages: List[Message],lang: str = 'zh',max_ref_token: int = 4000,**kwargs) -> Iterator[List[Message]]:"""Define the workflow"""assert isinstance(messages[-1]['content'], list) and any([item.image for item in messages[-1]['content']]), 'This agent requires input of images'# Image understandingnew_messages = copy.deepcopy(messages)new_messages[-1]['content'].append(ContentItem(text='Please provide a detailed description of all the details of this image'))response = []for rsp in self.image_agent.run(new_messages):yield response + rspresponse.extend(rsp)new_messages.extend(rsp)# Writing articlenew_messages.append(Message('user', 'Start writing your narrative essay based on the above image content!'))for rsp in self.writing_agent.run(new_messages,lang=lang,max_ref_token=max_ref_token,**kwargs):yield response + rsp
Demo2: 文档问答Agent,根据给定的参考资料来回答问题。
import copy
from typing import Iterator, Listfrom qwen_agent import Agent
from qwen_agent.llm.schema import CONTENT, ROLE, SYSTEM, MessagePROMPT_TEMPLATE_ZH = """
请充分理解以下参考资料内容,组织出满足用户提问的条理清晰的回复。
#参考资料:
{ref_doc}"""PROMPT_TEMPLATE_EN = """
Please fully understand the content of the following reference materials and organize a clear response that meets the user's questions.
# Reference materials:
{ref_doc}"""# 定义了两个语言的系统提示模板(中文和英文),用于引导模型回答问题
# PROMPT_TEMPLATE:根据语言类型返回相应模板
PROMPT_TEMPLATE = {'zh': PROMPT_TEMPLATE_ZH,'en': PROMPT_TEMPLATE_EN,
}class DocQA(Agent):# messages:用户与助理之间的消息列表。# knowledge:参考资料内容,作为系统提示模板的一部分。# lang:语言类型,默认为英文('en')。def _run(self,messages: List[Message],knowledge: str = '',lang: str = 'en',**kwargs) -> Iterator[List[Message]]:messages = copy.deepcopy(messages)system_prompt = PROMPT_TEMPLATE[lang].format(ref_doc=knowledge)if messages[0][ROLE] == SYSTEM:messages[0][CONTENT] += system_promptelse:messages.insert(0, Message(SYSTEM, system_prompt))return self._call_llm(messages=messages)
2-2、Tool
2-2-1、工具使用
1、工具统一使用.call(params)接口进行调用,您可以在其中为工具传递必要的参数。例如:直接的唤醒一个图片生成工具
from qwen_agent.tools import ImageGentool = ImageGen()
res = tool.call(params = {'prompt': 'a cute cat'})
print(res)
2-2-2、工具开发
Qwen-Agent提供了注册工具的机制。例如,要注册您自己的图片生成工具:
- 指定工具的名称、描述和参数。请注意,传递给@register_tool(‘my_image_gen’)的字符串会自动添加为类的.name属性,并将作为工具的唯一标识符。
- 实现call(…)函数。
如下为范例:
import urllib.parse
import json5
import json
from qwen_agent.tools.base import BaseTool, register_tool
# Add a custom tool named my_image_gen:
@register_tool('my_image_gen')
class MyImageGen(BaseTool):description = 'AI painting (image generation) service, input text description, and return the image URL drawn based on text information.'parameters = [{'name': 'prompt','type': 'string','description':'Detailed description of the desired image content, in English','required': True}]def call(self, params: str, **kwargs) -> str:prompt = json5.loads(params)['prompt']prompt = urllib.parse.quote(prompt)return json.dumps({'image_url': f'https://image.pollinations.ai/prompt/{prompt}'},ensure_ascii=False)
一旦注册了这些工具,就可以像上面提到的那样使用它们。
如果不希望使用注册方法,也可以直接定义工具类,然后将工具对象传递给Agent(未注册的工具不支持传递工具名称或配置文件)。
import urllib.parse
import json5
import json
from qwen_agent.tools.base import BaseToolclass MyImageGen(BaseTool):name = 'my_image_gen'description = 'AI painting (image generation) service, input text description, and return the image URL drawn based on text information.'parameters = [{'name': 'prompt','type': 'string','description':'Detailed description of the desired image content, in English','required': True}]def call(self, params: str, **kwargs) -> str:prompt = json5.loads(params)['prompt']prompt = urllib.parse.quote(prompt)return json.dumps({'image_url': f'https://image.pollinations.ai/prompt/{prompt}'},ensure_ascii=False)
2-3、LLM
目前,Qwen- agent提供了Qwen的DashScope API和OpenAI API,以及Qwen- vl的DashScope API的访问接口。两者都已经支持流式函数调用。
2-3-1、LLM使用
LLM使用get_chat_model(cfg: Optional[Dict] = None) -> BaseChatModel接口统一调用,传入的参数是LLM的配置文件。配置文件格式如下:
- model_type:对应于特定的LLM类,是LLM类的注册名,即唯一ID。当使用内置的DashScope和OpenAI API时,该参数可以省略。对于外部注册的LLM类,必须提供此参数来指定类。
- model:具体的模型名
- model_server:模型服务地址
- generate_cfg:模型生成的参数LLM类统一使用
LLM .chat(…)接口生成响应,支持消息列表、函数和其他参数的输入。
from qwen_agent.llm import get_chat_modelllm_cfg = {# Use the model service provided by DashScope:# 'model_type': 'qwen_dashscope','model': 'qwen-max','model_server': 'dashscope',# Use your own model service compatible with OpenAI API:# 'model': 'Qwen',# 'model_server': 'http://127.0.0.1:7905/v1',# (Optional) LLM hyper-paramters:'generate_cfg': {'top_p': 0.8}}
llm = get_chat_model(llm_cfg)
messages = [{'role': 'user','content': "What's the weather like in San Francisco?"
}]
functions = [{'name': 'get_current_weather','description': 'Get the current weather in a given location','parameters': {'type': 'object','properties': {'location': {'type': 'string','description':'The city and state, e.g. San Francisco, CA',},'unit': {'type': 'string','enum': ['celsius', 'fahrenheit']},},'required': ['location'],},
}]# The streaming output responses
responses = []
for responses in llm.chat(messages=messages,functions=functions,stream=True):print(responses)
2-3-2、LLM开发
Qwen-Agent提供llm注册机制。在LLM基类中,实现了统一的LLM .chat(…)接口。新注册的llm只需要实现三个特定的功能:
- 非流式的生成接口;
- 流式生成接口(如果LLM本身不支持流生成,非流结果可以包装到生成器中返回);
- 一个函数调用接口。
如果新注册的LLM不支持函数调用,它可以继承Qwen-Agent中实现的BaseFnCallModel类。这个类通过包装一个类似于ReAct的工具调用提示符,实现了基于通用会话接口的函数调用。
三、基于Qwen-Agent的案例分析
3-1、
3-2、
参考文章:
Qwen-Agent : GitHub官网.
Qwen-Agent 文档
总结
会调用工具的Agent太炫酷啦。🐏